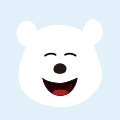
GraphRAG + Ollama 本地部署全攻略:避坑实战指南 原创
1、为什么要对 GraphRAG 本地部署?
微软开源 GraphRAG 后,热度越来越高,目前 GraphRAG 只支持 OpenAI 的闭源大模型,导致部署后使用范围大大受限,本文通过 GraphRAG 源码的修改,来支持更广泛的 Embedding 模型和开源大模型,从而使得 GraphRAG 的更容易上手使用。
2、GraphRAG 一键安装
第一步、安装 GraphRAG
需要 Python 3.10-3.12 环境。
第二步、创建知识数据文件夹
安装完整后,需要创建一个文件夹,用来存储你的知识数据,目前 GraphRAG 只支持 txt 和 csv 格式。
第三步、准备一份数据放在 /ragtest/input 目录下
第四步、初始化工作区
首先,我们需要运行以下命令来初始化。
其次,我们第二步已经准备了 ragtest 目录,运行以下命令完成初始化。
运行完成后,在 ragtest 目录下生成以下两个文件:.env
和settings.yaml
。ragtest 目录下的结构如下:
.env
文件包含了运行 GraphRAG 管道所需的环境变量。如果您检查该文件,您会看到一个定义的环境变量,GRAPHRAG_API_KEY=<API_KEY>
。这是 OpenAI API 或 Azure OpenAI 端点的 API 密钥。您可以用自己的 API 密钥替换它。
settings.yaml
文件包含了管道的设置。您可以修改此文件以更改管道的设置。
3、修改配置文件支持本地部署大模型
第一步、确保已安装 Ollama
如果你还没安装或者不会安装,可以参考我之前写的文章《Spring AI + Ollama 快速构建大模型应用程序(含源码)》。
第二步、确保已安装以下本地模型
Embedding 嵌入模型
quentinz/bge-large-zh-v1.5:latest
LLM 大模型
gemma2:9b
第三步、修改 settings.yaml 以支持以上两个本地模型,以下是修改后的文件
encoding_model: cl100k_base
skip_workflows: []
llm:
api_key: ollama
type: openai_chat # or azure_openai_chat
model: gemma2:9b # 你 ollama 中的本地 llm 模型,可以换成其他的,只要你安装了就可以
model_supports_json: true # recommended if this is available for your model.
max_tokens: 2048
api_base: http://localhost:11434/v1 # 接口注意是v1
concurrent_requests: 1 # the number of parallel inflight requests that may be made
parallelization:
stagger: 0.3
async_mode: threaded # or asyncio
embeddings:
async_mode: threaded # or asyncio
llm:
api_key: ollama
type: openai_embedding # or azure_openai_embedding
model: quentinz/bge-large-zh-v1.5:latest # 你 ollama 中的本地 Embeding 模型,可以换成其他的,只要你安装了就可以
api_base: http://localhost:11434/api # 注意是 api
concurrent_requests: 1 # the number of parallel inflight requests that may be made
chunks:
size: 300
overlap: 100
group_by_columns: [id] # by default, we don't allow chunks to cross documents
input:
type: file # or blob
file_type: text # or csv
base_dir: "input"
file_encoding: utf-8
file_pattern: ".*\\.txt$"
cache:
type: file # or blob
base_dir: "cache"
storage:
type: file # or blob
base_dir: "output/${timestamp}/artifacts"
reporting:
type: file # or console, blob
base_dir: "output/${timestamp}/reports"
entity_extraction:
prompt: "prompts/entity_extraction.txt"
entity_types: [organization,person,geo,event]
max_gleanings: 0
summarize_descriptions:
prompt: "prompts/summarize_descriptions.txt"
max_length: 500
claim_extraction:
prompt: "prompts/claim_extraction.txt"
description: "Any claims or facts that could be relevant to information discovery."
max_gleanings: 0
community_report:
prompt: "prompts/community_report.txt"
max_length: 2000
max_input_length: 8000
cluster_graph:
max_cluster_size: 10
embed_graph:
enabled: false # if true, will generate node2vec embeddings for nodes
umap:
enabled: false # if true, will generate UMAP embeddings for nodes
snapshots:
graphml: false
raw_entities: false
top_level_nodes: false
local_search:
max_tokens: 5000
global_search:
max_tokens: 5000
第四步、运行 GraphRAG 构建知识图谱索引
构建知识图谱的索引需要一定的时间,构建过程如下所示:
4、修改源码支持本地部署大模型
接下来修改源码,保证进行 local 和 global 查询时给出正确的结果。
第一步、修改成本地的 Embedding 模型
修改源代码的目录和文件:
.../Python/Python310/site-packages/graphrag/llm/openai/openai_embeddings_llm.py"
修改后的源码如下:
# Copyright (c) 2024 Microsoft Corporation.
# Licensed under the MIT License
"""The EmbeddingsLLM class."""
from typing_extensions import Unpack
from graphrag.llm.base import BaseLLM
from graphrag.llm.types import (
EmbeddingInput,
EmbeddingOutput,
LLMInput,
)
from .openai_configuration import OpenAIConfiguration
from .types import OpenAIClientTypes
import ollama
class OpenAIEmbeddingsLLM(BaseLLM[EmbeddingInput, EmbeddingOutput]):
"""A text-embedding generator LLM."""
_client: OpenAIClientTypes
_configuration: OpenAIConfiguration
def __init__(self, client: OpenAIClientTypes, configuration: OpenAIConfiguration):
self.client = client
self.configuration = configuration
async def _execute_llm(
self, input: EmbeddingInput, **kwargs: Unpack[LLMInput]
) -> EmbeddingOutput | None:
args = {
"model": self.configuration.model,
**(kwargs.get("model_parameters") or {}),
}
embedding_list = []
for inp in input:
embedding = ollama.embeddings(model="quentinz/bge-large-zh-v1.5:latest",prompt=inp)
embedding_list.append(embedding["embedding"])
return embedding_list
# embedding = await self.client.embeddings.create(
# input=input,
# **args,
# )
# return [d.embedding for d in embedding.data]
第二步、继续修改 Embedding 模型
修改源代码的目录和文件:
.../Python/Python310/site-packages/graphrag/query/llm/oai/embedding.py"
修改后的源码如下:
# Copyright (c) 2024 Microsoft Corporation.
# Licensed under the MIT License
"""OpenAI Embedding model implementation."""
import asyncio
from collections.abc import Callable
from typing import Any
import numpy as np
import tiktoken
from tenacity import (
AsyncRetrying,
RetryError,
Retrying,
retry_if_exception_type,
stop_after_attempt,
wait_exponential_jitter,
)
from graphrag.query.llm.base import BaseTextEmbedding
from graphrag.query.llm.oai.base import OpenAILLMImpl
from graphrag.query.llm.oai.typing import (
OPENAI_RETRY_ERROR_TYPES,
OpenaiApiType,
)
from graphrag.query.llm.text_utils import chunk_text
from graphrag.query.progress import StatusReporter
from langchain_community.embeddings import OllamaEmbeddings
class OpenAIEmbedding(BaseTextEmbedding, OpenAILLMImpl):
"""Wrapper for OpenAI Embedding models."""
def __init__(
self,
api_key: str | None = None,
azure_ad_token_provider: Callable | None = None,
model: str = "text-embedding-3-small",
deployment_name: str | None = None,
api_base: str | None = None,
api_version: str | None = None,
api_type: OpenaiApiType = OpenaiApiType.OpenAI,
organization: str | None = None,
encoding_name: str = "cl100k_base",
max_tokens: int = 8191,
max_retries: int = 10,
request_timeout: float = 180.0,
retry_error_types: tuple[type[BaseException]] = OPENAI_RETRY_ERROR_TYPES, # type: ignore
reporter: StatusReporter | None = None,
):
OpenAILLMImpl.__init__(
self=self,
api_key=api_key,
azure_ad_token_provider=azure_ad_token_provider,
deployment_name=deployment_name,
api_base=api_base,
api_versinotallow=api_version,
api_type=api_type, # type: ignore
organizatinotallow=organization,
max_retries=max_retries,
request_timeout=request_timeout,
reporter=reporter,
)
self.model = model
self.encoding_name = encoding_name
self.max_tokens = max_tokens
self.token_encoder = tiktoken.get_encoding(self.encoding_name)
self.retry_error_types = retry_error_types
def embed(self, text: str, **kwargs: Any) -> list[float]:
"""
Embed text using OpenAI Embedding's sync function.
For text longer than max_tokens, chunk texts into max_tokens, embed each chunk, then combine using weighted average.
Please refer to: https://github.com/openai/openai-cookbook/blob/main/examples/Embedding_long_inputs.ipynb
"""
token_chunks = chunk_text(
text=text, token_encoder=self.token_encoder, max_tokens=self.max_tokens
)
chunk_embeddings = []
chunk_lens = []
for chunk in token_chunks:
try:
embedding, chunk_len = self._embed_with_retry(chunk, **kwargs)
chunk_embeddings.append(embedding)
chunk_lens.append(chunk_len)
# TODO: catch a more specific exception
except Exception as e: # noqa BLE001
self._reporter.error(
message="Error embedding chunk",
details={self.__class__.__name__: str(e)},
)
continue
chunk_embeddings = np.average(chunk_embeddings, axis=0, weights=chunk_lens)
chunk_embeddings = chunk_embeddings / np.linalg.norm(chunk_embeddings)
return chunk_embeddings.tolist()
async def aembed(self, text: str, **kwargs: Any) -> list[float]:
"""
Embed text using OpenAI Embedding's async function.
For text longer than max_tokens, chunk texts into max_tokens, embed each chunk, then combine using weighted average.
"""
token_chunks = chunk_text(
text=text, token_encoder=self.token_encoder, max_tokens=self.max_tokens
)
chunk_embeddings = []
chunk_lens = []
embedding_results = await asyncio.gather(*[
self._aembed_with_retry(chunk, **kwargs) for chunk in token_chunks
])
embedding_results = [result for result in embedding_results if result[0]]
chunk_embeddings = [result[0] for result in embedding_results]
chunk_lens = [result[1] for result in embedding_results]
chunk_embeddings = np.average(chunk_embeddings, axis=0, weights=chunk_lens) # type: ignore
chunk_embeddings = chunk_embeddings / np.linalg.norm(chunk_embeddings)
return chunk_embeddings.tolist()
def _embed_with_retry(
self, text: str | tuple, **kwargs: Any
) -> tuple[list[float], int]:
try:
retryer = Retrying(
stop=stop_after_attempt(self.max_retries),
wait=wait_exponential_jitter(max=10),
reraise=True,
retry=retry_if_exception_type(self.retry_error_types),
)
for attempt in retryer:
with attempt:
embedding = (
OllamaEmbeddings(
model=self.model,
).embed_query(text)
or []
)
return (embedding, len(text))
except RetryError as e:
self._reporter.error(
message="Error at embed_with_retry()",
details={self.__class__.__name__: str(e)},
)
return ([], 0)
else:
# TODO: why not just throw in this case?
return ([], 0)
async def _aembed_with_retry(
self, text: str | tuple, **kwargs: Any
) -> tuple[list[float], int]:
try:
retryer = AsyncRetrying(
stop=stop_after_attempt(self.max_retries),
wait=wait_exponential_jitter(max=10),
reraise=True,
retry=retry_if_exception_type(self.retry_error_types),
)
async for attempt in retryer:
with attempt:
embedding = (
await OllamaEmbeddings(
model=self.model,
).embed_query(text) or [] )
return (embedding, len(text))
except RetryError as e:
self._reporter.error(
message="Error at embed_with_retry()",
details={self.__class__.__name__: str(e)},
)
return ([], 0)
else:
# TODO: why not just throw in this case?
return ([], 0)
5、GraphRAG 效果测试
第一、local 查询
第二、global 查询
本文转载自公众号玄姐聊AGI 作者:玄姐
原文链接:https://mp.weixin.qq.com/s/n7r627644R_q-chLFADfDw
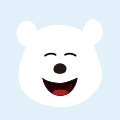