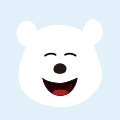
LlamaIndex结合DSPy,进一步优化RAG系统
本文带读者了解如何运用LlamaIndex和DSPy这两个工具来构建和优化检索增强型生成(Retrieval-Augmented Generation, RAG)系统。通过这两个框架的无缝结合,我们不仅能够简化开发过程,还能显著提高RAG系统的整体性能。
接下来,将详细解析LlamaIndex与DSPy如何高效协同,带来1+1>2的效果。
1 LlamaIndex
LlamaIndex 是用于构建、管理和查询大型语言模型(LLM)索引的开源工具。其主要目的是简化和优化与LLM 的交互过程,提供更高效的数据检索和组织方式,以优化用户体验。
LlamaIndex 就像图书馆,通过高效的索引构建和管理,将海量数据有序组织起来,使大型语言模型(LLMs)能迅速准确地检索信息。正如图书馆目录系统简化了书籍查找一样,LlamaIndex 同样简化了开发者与 LLMs 的交互,大幅提高了数据检索效率;LlamaIndex 还为开发者提供了简便易用的接口,是开发者的宝贵助手。
2 DSPy
DSPy 为大型语言模型(LLMs)的交互引入了一种创新的编程机制,取代了传统的手动提示编写。
通过定义 LLMs 的输入输出规范,DSPy能够自动定制出最适合特定应用场景的最优提示,从而提高交互效率,增强对不同场景的适应性,为开发者提供了一种更高效、灵活的交互手段。
3 RAG系统优化:LlamaIndex与DSPy的协同效益
LlamaIndex与DSPy的强强联合,为打造高效的RAG系统带来了一系列优势:
- 简化开发:DSPy摒弃了繁琐的手动提示编写,通过定义清晰的输入输出结构,自动化处理后续流程,极大地简化了开发工作。
- 提升性能:DSPy的智能优化功能能够确保每次交互都使用最合适的提示,从而带来更优越的性能和更准确的输出。
- 灵活性与可扩展性:LlamaIndex提供的丰富预构建模块,结合DSPy的高适应性,使RAG系统能够根据具体需求灵活定制,并随着业务的发展轻松扩展。
4 代码实现:构建RAG系统
LlamaIndex和DSPy提供了三种主要的集成方法,助力开发者搭建和优化RAG系统:
- 使用DSPy预测器优化查询流程构建:这种方法涉及编写DSPy代码来定义LLM输入输出规范。这些定义随后可以无缝地整合入LlamaIndex的查询流程,构建起一个完整的优化系统。
- 使用DSPy优化现有提示:无需从头编写DSPy代码,开发者可以直接设定LlamaIndex的提示模板,由系统内置的转换器自动运用DSPy的算法进行优化。
- DSPy优化提示在LlamaIndex模块中的应用:
DSPyPromptTemplate
模块作为桥梁,开发者可以将DSPy生成的优化提示应用于任何需要提示的LlamaIndex模块。
步骤I:安装库和下载数据
!pip install llama-index==0.10.44 git+https://github.com/stanfordnlp/dspy.git
# 下载数据
!wget https://raw.githubusercontent.com/run-llama/llama_index/main/docs/docs/examples/data/paul_graham/paul_graham_essay.txt -O paul_graham_essay.txt
步骤II:设置
import dspy
turbo = dspy.OpenAI(model='gpt-3.5-turbo')
dspy.settings.configure(lm=turbo)
class GenerateAnswer(dspy.Signature):
"""Answer questions with short factoid answers."""
context_str = dspy.InputField(desc="contains relevant facts")
query_str = dspy.InputField()
answer = dspy.OutputField(desc="often between 1 and 5 words")
步骤III:构建索引
from llama_index.core import SimpleDirectoryReader, VectorStoreIndex
reader = SimpleDirectoryReader(input_files=["paul_graham_essay.txt"])
docs = reader.load_data()
index = VectorStoreIndex.from_documents(docs)
retriever = index.as_retriever(similarity_top_k=2)
步骤IV:构建查询管道
from llama_index.core.query_pipeline import QueryPipeline as QP, InputComponent, FnComponent
from dspy.predict.llamaindex import DSPyComponent, LlamaIndexModule
dspy_component = DSPyComponent(
dspy.ChainOfThought(GenerateAnswer)
)
retriever_post = FnComponent(
lambda contexts: "\n\n".join([n.get_content() for n in contexts])
)
p = QP(verbose=True)
p.add_modules(
{
"input": InputComponent(),
"retriever": retriever,
"retriever_post": retriever_post,
"synthesizer": dspy_component,
}
)
p.add_link("input", "retriever")
p.add_link("retriever", "retriever_post")
p.add_link("input", "synthesizer", dest_key="query_str")
p.add_link("retriever_post", "synthesizer", dest_key="context_str")
dspy_qp = LlamaIndexModule(p)
output = dspy_qp(query_str="what did the author do in YC")
# 输出
Prediction(
answer='Worked with startups, funded them.'
)
5 结语
LlamaIndex和DSPy的集成为开发高效能的RAG系统开启了新的篇章。
这一集成充分发挥了两个框架的互补优势,使开发者得以借助自动化的提示优化技术、简化的开发流程,以及丰富的预构建模块库,打造出更为复杂且具有深远影响力的RAG解决方案。这不仅提升了系统的综合性能,也为多样化应用场景中的RAG系统开发提供了坚实基础。
本文转载自 AI科技论谈,作者: AI科技论谈
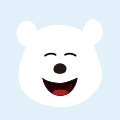